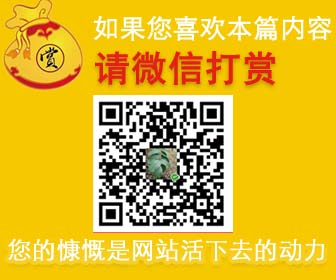
针对在数据不平衡的情况下,异常类的准确率急剧下降的问题,前人已经做了很多研究,并从不同角度提出了多种解决方案,包括样例采样[9-10]、样例加权(也称代价敏感学习)[11]、分类面偏移[12]、一类分类器[13]以及集成学习[14-15]等。就ELM而言,Zong等[16]提出的加权极限学习机(WeightedExtremeLearningMachine,WELM),通过给样本数量处于劣势的少数类样本赋予高权重,从而提高分类的准确率。但是这种算法的缺点也显而易见:(1)该算法给同一类样本赋予相等的权重,这就忽视了同一类不同样本之间的分布,众所周知,如果一个样本的周围有很多同类样本,则该样本比较重要;反之,如果一个样本周围没有样本,或者有很多异类样本,则该样本点是离群点或噪声点,应该赋予一个较小的权重。(2)如果数据的不平衡比例过大,则很难调整过来。比如,不平衡比例很大的数据集,其分类面通常严重偏移,使得多数类和少数类样本出现在同一侧论文网,这样通过WELM调节后的分类面很可能只是向少数类偏移了一点,其偏移量可能是远远不够的。80846
具体到采样技术,主要有以下两种:欠采样和过采样,欠采样是通过去掉多数类的一些样本来实现平衡的,过采样则通过增加一些少数类样本使得两类样本的数量均衡。Vong等[17]将ELM和随机过采样技术(RandomOverSampling,ROS)相结合并用于空气质量检测,提升了空气中固体颗粒物等级的识别率;Sun等[18]则将SMOTE(SyntheticMinorityOversamplingTEchnique)算法[19]引入到ELM集成学习的框架中去,在企业生命周期的预测任务上获得了很好的性能。
参考文献
[1] Hilbert, Martin。 The World's Technological Capacity to Store, Communicate, and Compute Information。 Science, 2011, 332 (6025): 60–65
[2] McAfee A, Brynjolfsson E, Davenport T H, et al。 Big data。 The management revolution。 Harvard Bus Rev, 2012, 90(10): 61-67
[3] Community cleverness required。 Nature, 4 September 2008, 455 (7209):1
[4] Huang G B, Zhu Q Y, Siew C K。 Extreme learning machine: theory and applications。
Neurocomputing, 2006, 70: 489-501
[5] Rumelhart D E, Hinton G E, Williams R J。 Learning representations by back- propagation errors [J]。 Nature, 1986, 323: 533-536
[6] Huang G B, Zhou H, Ding X, et al。 Extreme learning machine for regression and multiclass classification [J]。 IEEE Transactions on System, Man and Cybernetics, Part B:
Cybernetics, 2012, 42: 513-529
[7] Huang G, Huang G B, Song S, et al。 Trends in Extreme Learning Machine: A Review,
Neural Networks [J]。 2015, 61: 32-48
[8] Zong W, Huang G B, Chen Y。 Weighted extreme learning machine for imbalance learning [J]。 Neurocomputing, 2013, 101: 229-242
[9] Chawla N V, Bowyer K W, Hall L O。 SMOTE: Synthetic Minority Over-Sampling Technique [J]。 Journal of Artificial Intelligence Research, 2002, 16: 321-357
[10] Zeng Zhi qiang, Wu Qun, Liao Bei shui, et al。 A Classification Method for Imbalance Data Set Based on Kernel SMOTE [J]。 ACTA ELECTRONICA SINICA, 2009, 37(11):
2489-2495(in Chinese)
[11] Batuwita R, Palade V。 FSVM-CIL: Fuzzy Support Vector Machines for Class Imbalance Learning [J]。 IEEE Transactions on Fuzzy Systems, 2010, 18: 558-571
[12] Yu H, Mu C, Sun C, et al。 Support Vector Machine-Based Optimized Decision Threshold Adjustment Strategy for Classifying Imbalanced Data [J]。 Knowledge-Based Systems, 2015, 76: 67-78
[13] Maldonado S, Montecinos C。 Robust classification of imbalanced data using one-class and two-class SVM-based multiclassifiers [J]。 Intelligent Data Analysis, 2014, 18: 95-112。 [14] Yu H, Ni J。 An Improved Ensemble Learning Method for Classifying High- dimensional and Imbalanced Biomedicine Data [J]。 IEEE/ACM Transactions on Computational Biology and Bioinformatics, 2014, 11: 657-666 加权极限学习机研究现状和参考文献:http://www.youerw.com/yanjiu/lunwen_94101.html