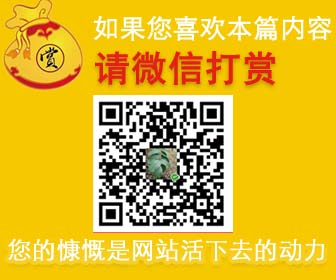
[15] Park Y, Ghosh J。 Ensembles of α-trees for imbalanced classification problems [J]。
IEEE Transactions on Knowledge and Data Engineering, 2014, 26: 131-143
[16] Zong W, Huang G B, Chen Y。 Weighted extreme learning machine for imbalance learning [J]。 Neurocomputing, 2013, 101: 229-242
[17] Sun S J, Chang C, Hsu M F。 Multiple extreme learning machines for a two-class imbalance corporate life cycle prediction [J]。 Knowledge-Based Systems, 2013, 39: 214- 223
[18] Wan C, Xu Z, Pinson P, et al。 Probabilistic Forecasting of Wind Power Generation Using Extreme Learning Machine [J]。 IEEE Transactions on Power Systems, 2014, 29: 1033-1044。
[19] Chawla N V, Bowyer K W, Hall L O。 SMOTE: Synthetic Minority Over-Sampling Technique [J]。 Journal of Artificial Intelligence Research, 2002, 16: 321-357
[20] Japkowicz N。 Learning from imbalanced data sets: a comparison of various strategies[C]。 AAAI workshop on learning from imbalanced data sets。 2000, 68: 10-15
[21] Chawla N V, Japkowicz N, Kotcz A。 Editorial: special issue on learning from imbalanced data sets[J]。 ACM Sigkdd Explorations Newsletter, 2004, 6(1): 1-6
[22] Chawla N。 Mining when classes are imbalanced, rare events matter more, and errors have costs attached[C]。 Proc。 of SDM。 2009
[23] Ling C X, Li C。 Data Mining for Direct Marketing: Problems and Solutions[C]。 KDD。 1998, 98: 73-79
[24] Chawla N V, Bowyer K W, Hall L O, et al。 SMOTE: synthetic minority over- sampling technique[J]。 Journal of artificial intelligence research, 2002: 321-357
[25] Hussein, El-Saadi。 Informed Under-Sampling for Enhancing Patient Specific Epileptic Seizure Detection[J]。 International Journal of Computer Applications (0975 – 8887) , 2012, 57(16): 1-6
[26] Veropoulos K, Campbell C, Cristianini N。 Controlling the sensitivity of support vector machines[C]。 Proceedings of the international joint conference on AI。 1999: 55-60
[27] Zhou Z H, Liu X Y。 Training cost-sensitive neural networks with methods addressing the class imbalance problem[J]。 Knowledge and Data Engineering。 IEEE Transactions on, 2006, 18(1): 63-77
[28] W。 Zong, G。 B。 Huang, Y。 Chen。 Weighted extreme learning machine for imbalance learning, Neurocomputing, 101 (2013): 229-242
[29] R。 Batuwita, V。 Palade。 FSVM-CIL: Fuzzy Support Vector Machines for Class
Imbalance Learning。 IEEE Trans。 Fuzzy Syst。, 18 (2010): 558-571。
[30] Lin W J, Chen J J。 Class-imbalanced classifiers for high-dimensional data[J]。
Briefings in bioinformatics, 2013, 14(1): 13-26
[31] Yu H, Mu C, Sun C, et al。 Support vector machine-based optimized decision threshold adjustment strategy for classifying imbalanced data[J]。 Knowledge-Based Systems, 2015, 76: 67-78
[32] Yu H, Sun C, Yang X, et al。 ODOC-ELM: Optimal decision outputs compensation- based extreme learning machine for classifying imbalanced data[J]。 Knowledge-Based Systems, 2016, 92: 55-70
[33] 潘志松,陈斌,缪志敏,等。 One-Class 分类器研究。 电子学报, 37 (2009): 2496-2503
[34] Mena L, Gonzalez J A。 Symbolic one-class learning from imbalanced datasets: application in medical diagnosis[J]。 International Journal on Artificial Intelligence Tools, 2009, 18(02): 273-309
[35] Maldonado S, Montecinos C。 Robust classification of imbalanced data using one-class and two-class SVM-based multiclassifiers[J]。 Intelligent Data Analysis, 2014, 18(1): 95- 112
[36] Chawla N V, Lazarevic A, Hall L O, et al。 SMOTEBoost: Improving prediction of the minority class in boosting[M]//Knowledge Discovery in Databases: PKDD 2003。 Springer Berlin Heidelberg, 2003: 107-119 加权极限学习机研究现状和参考文献(2):http://www.youerw.com/yanjiu/lunwen_94101.html